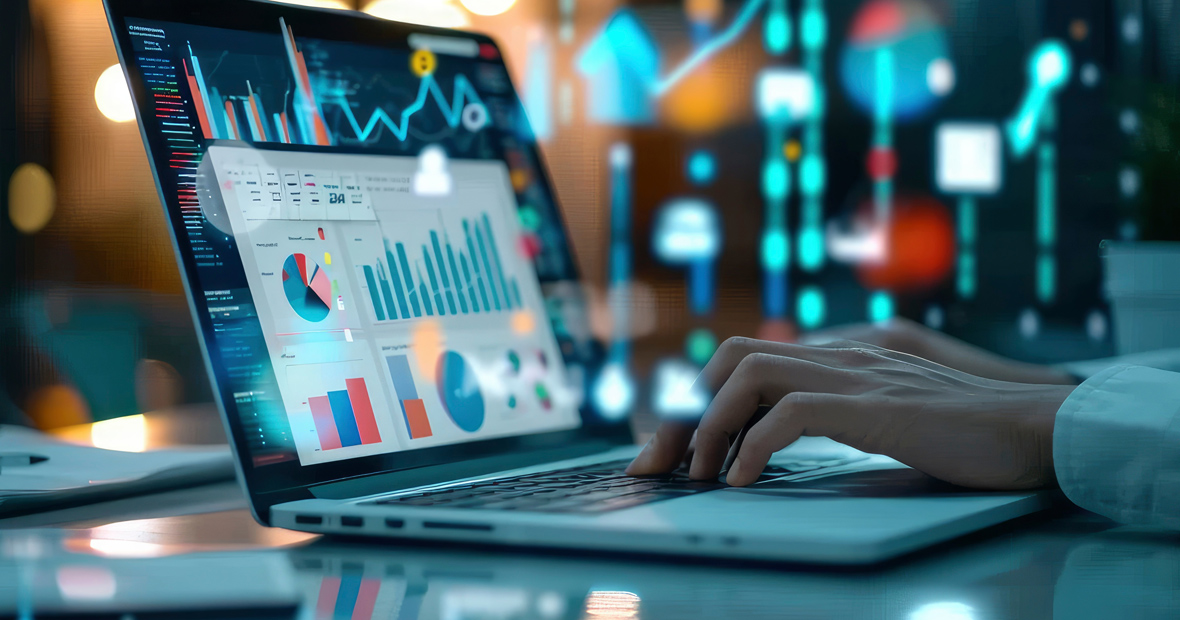
Data analytics has emerged as an indispensable tool for organizations seeking to enhance their risk management capabilities. The proliferation of big data and advancements in analytical technologies have enabled businesses to gain deeper insights into potential threats and opportunities. By leveraging data-driven approaches, companies can identify, assess, and mitigate risks more effectively across various operational domains. The integration of analytics into risk management frameworks allows for more proactive and informed decision-making. This approach facilitates a comprehensive understanding of risk factors, empowering organizations to develop robust strategies for risk mitigation and operational resilience.
Key Steps in Data-Driven Risk Management Process
The data-driven risk management process encompasses several interconnected steps that form the foundation for effective risk identification, assessment, and mitigation. This systematic approach harnesses the power of data analytics to provide organizations with a comprehensive view of their risk landscape. By following these steps, businesses can develop a more nuanced understanding of potential threats and opportunities, enabling them to make informed decisions and allocate resources more effectively. Get more details by visiting Axians website.
Identifying Potential Risks Across Business Functions
The initial phase of data-driven risk management involves a thorough examination of potential risks across various business functions. This process requires a systematic approach to identify and categorize risks that may impact the organization's operations, financial performance, or strategic objectives. Data analytics tools can be employed to analyze historical data, industry trends, and external factors to uncover patterns and potential risk indicators. By leveraging machine learning algorithms, organizations can detect anomalies and emerging risks that may not be immediately apparent through traditional risk assessment methods.
Risk identification should encompass a wide range of potential threats, such as operational risks, financial risks, compliance risks, and strategic risks. Organizations can utilize data mining techniques to extract relevant information from internal databases, external sources, and industry reports. Advanced text analytics can be applied to unstructured data sources, such as social media feeds and customer feedback, to identify potential reputational risks or emerging market trends that may pose threats to the business. By integrating data from multiple sources, organizations can create a comprehensive risk profile that considers both internal and external factors.
Gathering Relevant Data from Multiple Sources
Effective risk management relies on the collection and integration of relevant data from diverse sources. Organizations must develop robust data gathering strategies that encompass both internal and external data points. Internal data sources may include financial records, operational metrics, employee performance data, and customer information. External data sources can encompass market trends, regulatory updates, economic indicators, and industry benchmarks. The challenge lies in aggregating and harmonizing data from these disparate sources to create a unified view of the organization's risk profile.
Data integration platforms and advanced ETL (Extract, Transform, Load) processes play a pivotal role in consolidating data from multiple sources. These tools enable organizations to standardize data formats, resolve inconsistencies, and create a centralized data repository for risk analysis. Implementation of data governance frameworks ensures data quality, accuracy, and consistency across the organization. This approach facilitates more reliable risk assessments and enables stakeholders to make informed decisions based on trustworthy data.
Organizations should also consider leveraging alternative data sources to enhance their risk management capabilities. This may involve incorporating data from IoT devices, satellite imagery, or social media sentiment analysis. By expanding the scope of data collection, businesses can gain a more comprehensive understanding of potential risks and opportunities. However, it is crucial to balance the breadth of data collection with considerations of data privacy, security, and regulatory compliance. Implementing robust data protection measures and adhering to relevant data protection regulations are essential components of a responsible data gathering strategy.
Analyzing Data to Assess Risk Likelihood
Once relevant data has been collected and integrated, the next step in the data-driven risk management process involves analyzing this information to assess the likelihood and potential impact of identified risks. Advanced statistical techniques and machine learning algorithms can be employed to analyze historical data and identify patterns that may indicate increased risk likelihood. Regression analysis, time series forecasting, and Monte Carlo simulations are among the analytical tools that can be used to quantify risk probabilities and potential financial impacts.
Predictive analytics models play a significant role in assessing risk likelihood. These models leverage historical data and current trends to forecast potential future scenarios. By utilizing techniques such as decision trees, neural networks, or ensemble methods, organizations can develop sophisticated risk prediction models that account for multiple variables and complex interactions. These models can be continuously refined and updated as new data becomes available, ensuring that risk assessments remain relevant and accurate in dynamic business environments.
The analysis of risk likelihood should also consider the potential correlation between different risk factors. Data analytics techniques such as cluster analysis and network analysis can help identify relationships between various risk elements, allowing organizations to develop a more nuanced understanding of their overall risk exposure. This approach enables businesses to prioritize risks more effectively and allocate resources to areas that pose the greatest potential threat. Additionally, sensitivity analysis can be conducted to assess how changes in key variables may impact overall risk profiles, providing valuable insights for scenario planning and risk mitigation strategies.
Essential Data Analytics Tools for Risk Mitigation
The field of data analytics offers a wide array of tools and technologies that can be leveraged for effective risk mitigation. These tools enable organizations to process vast amounts of data, uncover hidden patterns, and generate actionable insights for risk management. By employing advanced analytics techniques, businesses can enhance their ability to identify, assess, and respond to potential risks in a timely manner. The integration of these tools into risk management frameworks can significantly improve an organization's overall risk posture and decision-making capabilities.
Predictive Analytics Models to Forecast Risks
Predictive analytics models represent a powerful tool in the risk management arsenal, allowing organizations to anticipate potential risks before they materialize. These models utilize historical data, current trends, and external factors to forecast future risk scenarios. By employing techniques such as regression analysis, time series forecasting, and machine learning algorithms, organizations can develop sophisticated risk prediction models that account for multiple variables and complex interactions. The output of these models can be used to inform strategic decision-making, resource allocation, and the development of proactive risk mitigation strategies.
One of the primary advantages of predictive analytics in risk management is the ability to conduct scenario analysis and stress testing. By simulating various potential outcomes, organizations can assess their resilience to different risk scenarios and identify potential vulnerabilities in their operations or financial structures. For example, financial institutions can use predictive models to assess the impact of economic downturns on their loan portfolios, enabling them to adjust their lending strategies and capital reserves accordingly. Similarly, supply chain managers can utilize predictive analytics to forecast potential disruptions and develop contingency plans to mitigate their impact.
The effectiveness of predictive analytics models in risk management can be enhanced through the integration of machine learning techniques. Algorithms such as random forests, gradient boosting machines, and deep learning neural networks can be employed to improve the accuracy and robustness of risk forecasts. These advanced techniques can capture non-linear relationships and complex patterns in data that may not be apparent through traditional statistical methods. Moreover, the use of ensemble methods, which combine multiple predictive models, can further improve forecast accuracy and provide a more comprehensive view of potential risks.
Data Visualization Dashboards for Risk Monitoring
Data visualization dashboards serve as a critical tool for effective risk monitoring and communication. These interactive platforms transform complex data sets into easily understandable visual representations, enabling stakeholders to quickly grasp key risk indicators and trends. By presenting risk-related information in a visually appealing and intuitive format, data visualization dashboards facilitate more efficient decision-making and foster a culture of risk awareness throughout the organization. The ability to customize dashboards for different user groups ensures that relevant information is readily accessible to those who need it most.
Advanced data visualization techniques can be employed to create dynamic risk heat maps, which provide a color-coded representation of risk levels across various dimensions. These heat maps allow stakeholders to quickly identify high-risk areas and allocate resources accordingly. Interactive features such as drill-down capabilities enable users to explore underlying data and gain deeper insights into specific risk factors. Additionally, the integration of real-time data feeds into visualization dashboards ensures that risk monitoring remains up-to-date and responsive to changing conditions.
The effectiveness of data visualization dashboards in risk management can be further enhanced through the incorporation of advanced analytics capabilities. For example, the integration of anomaly detection algorithms can automatically highlight unusual patterns or outliers in risk data, drawing attention to potential areas of concern. Predictive analytics can be incorporated to display forecasted risk trends alongside historical data, providing a forward-looking perspective on potential threats. Moreover, the use of natural language generation techniques can automatically generate narrative summaries of key risk insights, facilitating easier interpretation and communication of complex risk information.
AI Algorithms for Real-Time Risk Detection
Artificial Intelligence (AI) algorithms have revolutionized the field of risk management by enabling real-time risk detection and response. These advanced algorithms can process vast amounts of structured and unstructured data at high speeds, identifying potential risks and anomalies as they emerge. Machine learning techniques such as unsupervised learning and deep learning can be employed to detect patterns and relationships in data that may not be apparent through traditional analysis methods. This capability allows organizations to identify emerging risks and respond proactively, potentially averting crises before they escalate.
One of the primary applications of AI in risk detection is the identification of fraudulent activities. AI-powered fraud detection systems can analyze transaction data in real-time, flagging suspicious patterns or behaviors that may indicate fraudulent activity. These systems can learn from historical fraud cases and continuously adapt to new fraud techniques, improving their detection accuracy over time. Similarly, AI algorithms can be employed in cybersecurity applications to detect and respond to potential threats in real-time, enhancing an organization's ability to protect its digital assets and sensitive information.
Natural Language Processing (NLP) techniques represent another powerful application of AI in risk detection. These algorithms can analyze unstructured text data from sources such as news articles, social media posts, and customer feedback to identify potential reputational risks or emerging market trends. By processing and interpreting large volumes of textual data in real-time, NLP algorithms can provide early warning signals of potential risks, allowing organizations to take preemptive action. Moreover, the integration of sentiment analysis can help gauge public perception and identify potential shifts in stakeholder attitudes that may pose risks to the organization.
Integrating Data Analytics into Risk Management Framework
The integration of data analytics into existing risk management frameworks represents a significant shift in how organizations approach risk assessment and mitigation. This process involves aligning analytical capabilities with established risk management practices to create a more data-driven and proactive approach to managing risks. The successful integration of data analytics requires a comprehensive strategy that addresses technological, organizational, and cultural aspects of risk management. By embedding analytics throughout the risk management lifecycle, organizations can enhance their ability to identify, assess, and respond to risks in a more timely and effective manner.
One of the primary challenges in integrating data analytics into risk management frameworks is ensuring seamless data flow between various systems and processes. This may involve implementing data integration platforms that can consolidate information from disparate sources, creating a unified view of the organization's risk landscape. The development of standardized data models and taxonomies is crucial for ensuring consistency in risk reporting and analysis across different business units. Additionally, organizations must establish clear governance structures and data management policies to ensure the quality, security, and ethical use of data in risk management processes.
The integration of data analytics also necessitates a shift in organizational culture and mindset. Risk management professionals must be equipped with the necessary skills and knowledge to leverage analytical tools effectively. This may involve providing training programs in data analysis, statistical methods, and data visualization techniques. Moreover, fostering a data-driven culture throughout the organization is essential for maximizing the value of analytics in risk management. This cultural shift encourages employees at all levels to consider data and analytical insights in their decision-making processes, leading to more informed and objective risk assessments.
To facilitate the seamless integration of data analytics into risk management frameworks, organizations should consider adopting agile methodologies and iterative approaches. This allows for the gradual implementation of analytical capabilities, starting with pilot projects in specific risk domains and gradually expanding to cover broader areas of risk management. Regular evaluation and refinement of analytical models and processes ensure that they remain aligned with the organization's evolving risk management needs. Furthermore, the establishment of cross-functional teams that bring together risk management professionals, data scientists, and IT specialists can foster collaboration and drive innovation in risk analytics.
Best Practices for Data-Powered Risk Management Strategy
Developing and implementing a data-powered risk management strategy requires a thoughtful and systematic approach. Organizations must adopt best practices that ensure the effective use of data analytics while maintaining the integrity and reliability of their risk management processes. These best practices encompass various aspects of data management, analytical methodologies, and organizational alignment. By adhering to these guidelines, businesses can maximize the value of their data analytics initiatives and create a more robust and responsive risk management framework.
Defining Clear Risk Metrics Data Requirements
The foundation of an effective data-powered risk management strategy lies in defining clear and comprehensive risk metrics and data requirements. This process involves identifying the key risk indicators (KRIs) that are most relevant to the organization's strategic objectives and operational processes. By establishing a well-defined set of risk metrics, organizations can ensure that their data collection and analysis efforts are focused on the most critical aspects of their risk landscape. These metrics should be aligned with the organization's risk appetite and tolerance levels, providing a clear framework for risk assessment and decision-making.
When defining risk metrics and data requirements, it is essential to consider both quantitative and qualitative indicators. Quantitative metrics may include financial ratios, operational performance indicators, or market risk measures. Qualitative indicators could encompass factors such as compliance status, reputational assessments, or strategic alignment. The selection of appropriate metrics should be based on their relevance, measurability, and actionability. Additionally, organizations should consider the frequency of data collection and reporting for each metric, ensuring that the information remains timely and relevant for decision-making purposes.
Ensuring Data Quality Accuracy Completeness Timeliness
The reliability and effectiveness of data-powered risk management strategies hinge on the quality, accuracy, completeness, and timeliness of the underlying data. Organizations must implement robust data quality management processes to ensure that the information used for risk assessment and decision-making is trustworthy and fit for purpose. This involves establishing clear data quality standards, implementing data validation and cleansing procedures, and conducting regular audits to identify and address any data quality issues.
Data accuracy is paramount in risk management, as even small errors or inconsistencies can lead to flawed risk assessments and potentially costly decisions. Organizations should implement automated data validation checks to identify and flag potential errors or anomalies in incoming data. These checks may include range validation, consistency checks, and cross-referencing with other data sources. Additionally, implementing data lineage tracking can help organizations trace the origin and transformations of data, facilitating easier identification and resolution of data quality issues.
Continuously Refining Analytics Based on Results
The effectiveness of data-powered risk management strategies relies on the continuous refinement and improvement of analytical models and processes. Organizations must adopt an iterative approach to risk analytics, regularly evaluating the performance of their models and adapting them based on observed results and changing conditions. This process of continuous improvement ensures that risk management practices remain relevant and effective in the face of evolving business environments and emerging risks.
One key aspect of refining analytics is the regular validation and backtesting of predictive models. By comparing model predictions with actual outcomes, organizations can assess the accuracy and reliability of their risk forecasts. This process can help identify areas where models may be underperforming or failing to capture important risk factors. Based on these insights, analysts can refine model parameters, incorporate new variables, or explore alternative modeling techniques to improve predictive accuracy.